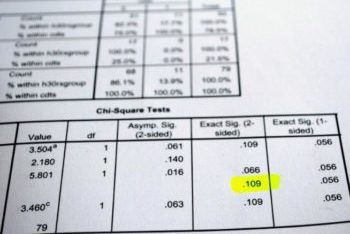
The P-Value is probably one of the most mis-interpreted areas of research. Not surprisingly, because our brains naturally try to impose the most simplistic heuristic to efficiently organize information. So yeah, it’s our brain’s fault!
There are two very interrelated concepts surrounding P-Values. The first is the concept is effect size. For example, an Odds Ratio of 2.0 is a stronger effect than an odd’s ratio of 1, which is about 50:50 in the probably of an event occurring in the presence of a covariate (Say males versus females). This is where the confusion lies. Standardized effect sizes are difficult to interpret, largely because of their scale. An effect of moving from 1.0 to 1.5 is not the same as moving from 100 to 150 when looking at something like IQ. Yet on some laboratory tests, going from 6.5 to 7.5 can indicate the difference between pre-diabetes and a diagnosis of diabetes. Instead of taking the time to understand the reference point, it is just easier to say that the Odds Ratio was highly significant, P<.001 and leave it at that!
The true conundrum is that you can have a small effect size highly significant, but a large effect size that is non-significant. The P-Value is really a measure of the strength of the inference about the effect. But it is not a direct measure of the effect’s strength. You can think of it this way, it is the probably of finding a significant effect when the finding is not true if the study were repeated many times.
So how is a P-Value related to a study’s effect size? This part is where a study’s sample size and the homogeneity of the population comes into play. The variance is a measure of dispersion around a measure, say the mean socioeconomic status of a school. The two means can be the same, but the variance around those means can be different. This can occur when one goes into a school that may be relatively homogenous, say a rural school, compared to a suburban school that busses students from an inner city, disadvantaged neighborhood. The means may be the same, but the variability in the population is so much greater in the suburban school than the rural school. As a side note, it is this very reason examine the study’s descriptive statistics, such as mean, variance, standardized variance (standard error/standard deviation) before diving deeper into a study’s findings. Editor’s Note: Do you like the way we wove in our Blog Theme here? Thanks to Mrs. Dombrowski’s 8th grade English composition class!
Here’s where the magic of statistics comes into the fold. Statistical significance can be quickly identified by visually inspecting the standard error bars around 2 separate factors. If they overlap, then there is likely to be no statistically significant effect. If they do not, then we can assume that both come from an independent population and differ significantly from one another.
Editor’s Note (again): Sorry to keep butting in, but there is a hook. Non-overlapping confidence intervals (Standard Errors) are statistically significant. But, there are rare occasions when you can get overlapping Confidence Intervals actually statistically significant. This is because the confidence intervals around the statistical tests are calculated slightly differently than the calculations for the observed mean and variance. Ok, back to the story…
Here’s the trick. It is possible to have the same mean and variance move from statistical non-significance to statistical significance merely by increasing the sample size. This process means we can shorten the width of the standard errors around the test statistic, decreasing the probability value of finding a no effect when one is present. But, if you had a trial of say 30 participants and the p-value was non-significant, you cannot under any circumstances state the same trial would have been significant if the trial recruited another 50 patients. The reason is that you cannot guarantee that the additional 50 patients will respond in the same manner as the first 30!
Editor’s Note: Me again (Jaki). Just also wanted to clarify what Scot was mentioning. Your hypotheses can be stated in many ways. You can hypothesize that the means of two factors in a variable are significantly different (2-tailed), that one is greater than the other (1-tailed), or equivalence (let’s deal with that one later). Often, we hypothesize a two tailed test (means are different) so we can detect an effect in either direction. This is more conservative than a 1 tailed tail test and is the norm in clinical research.
So hopefully you are not confused. But let me introduce one last point. There is a big difference between clinical significance and statistical significance. The latter of which is agnostic to the meaning of the data. In epidemiology, we can see there was a statistically significance change in the population of smokers by .001%, but that may translate to 225,000 persons if we assume the U.S. population to be around 255,000,000. While.001% may not seem big, it is large once you consider the raw numbers. Conversely, you may find that a drug statistically increases body temp by 0.1 degree, which clinically occurs when someone walks up a flight of stairs.
In conclusion, (Do you like the way I set that up!) we hope you now understand what exactly a P-Value represents and why you should avoid discussing it as a measure of an effect size. We like to think about it as how much confidence we can place in our actual finding. I can ask Jaki what the effect size was, and she can tell me it is an Odds Ratio of 2.0, or that adding bacon to my diet will nearly double my risk of heart disease. Wow—that seems like a lot, but then I ask her how confident in the finding, and then she tells me she has either strong confidence (P<.001), or moderately strong confidence (p=.049) in the finding. The level of confidence changes depending upon which covariates (confounders) are added.
The Bottom Line: Our team has tremendous experience in working with both “newbies” to statistics and those who are experience. As former college professors, we enjoy answering all your questions and unlike lawyers, we don’t charge per question! And speaking of school—-Van Halen Rulz!
Contact us at: 866-853-7828 or info@kingfishstat.com